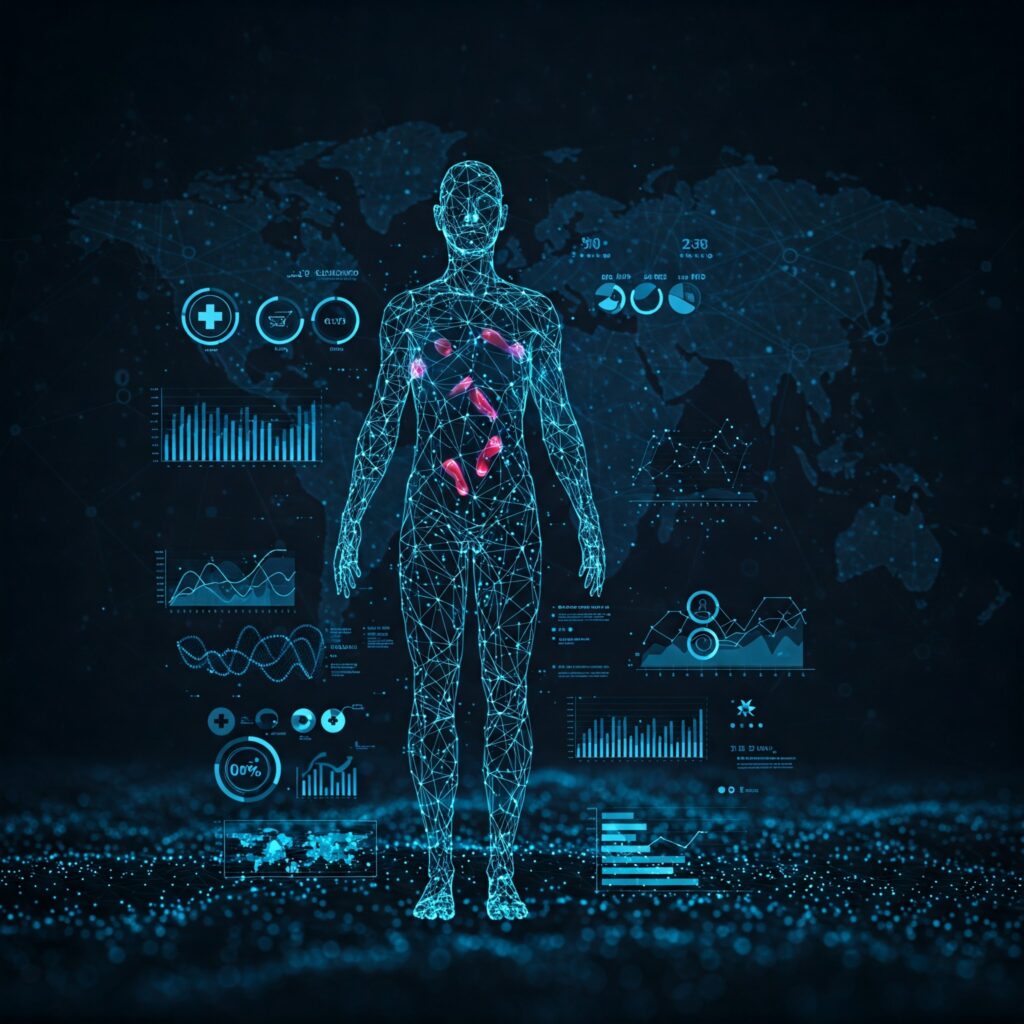
Health data is at the core of modern healthcare, enabling patient care, medical research, and public health policy. As healthcare services become increasingly digital, the volume and diversity of medical data have grown exponentially. This data is also crucial for training and validating artificial intelligence algorithms in healthcare, enabling predictive analytics, personalized medicine, and more effective disease management.
However, managing and securing this data requires standardized frameworks to ensure accuracy, interoperability, and patient privacy. This blog explores the types of health data, global data standards, and key security frameworks governing medical information.
AI-driven healthcare solutions depend on high-quality health data for training and validation. Key areas where AI leverages medical data include:
1. Predictive Analytics for Early Disease Detection
AI models trained on electronic health records (EHRs), lab results, and wearable device data can predict early signs of chronic diseases such as diabetes, cardiovascular disorders, and cancer. By analyzing patterns and trends in patient data, AI can identify at-risk individuals before symptoms become severe, enabling timely interventions and potentially saving lives.
Example: Apollo Hospitals in India uses AI-powered analytics to assess a patient’s health risks based on medical history, enabling early interventions for conditions like heart disease. This proactive approach helps in managing patient health more effectively and reducing the burden on healthcare systems.
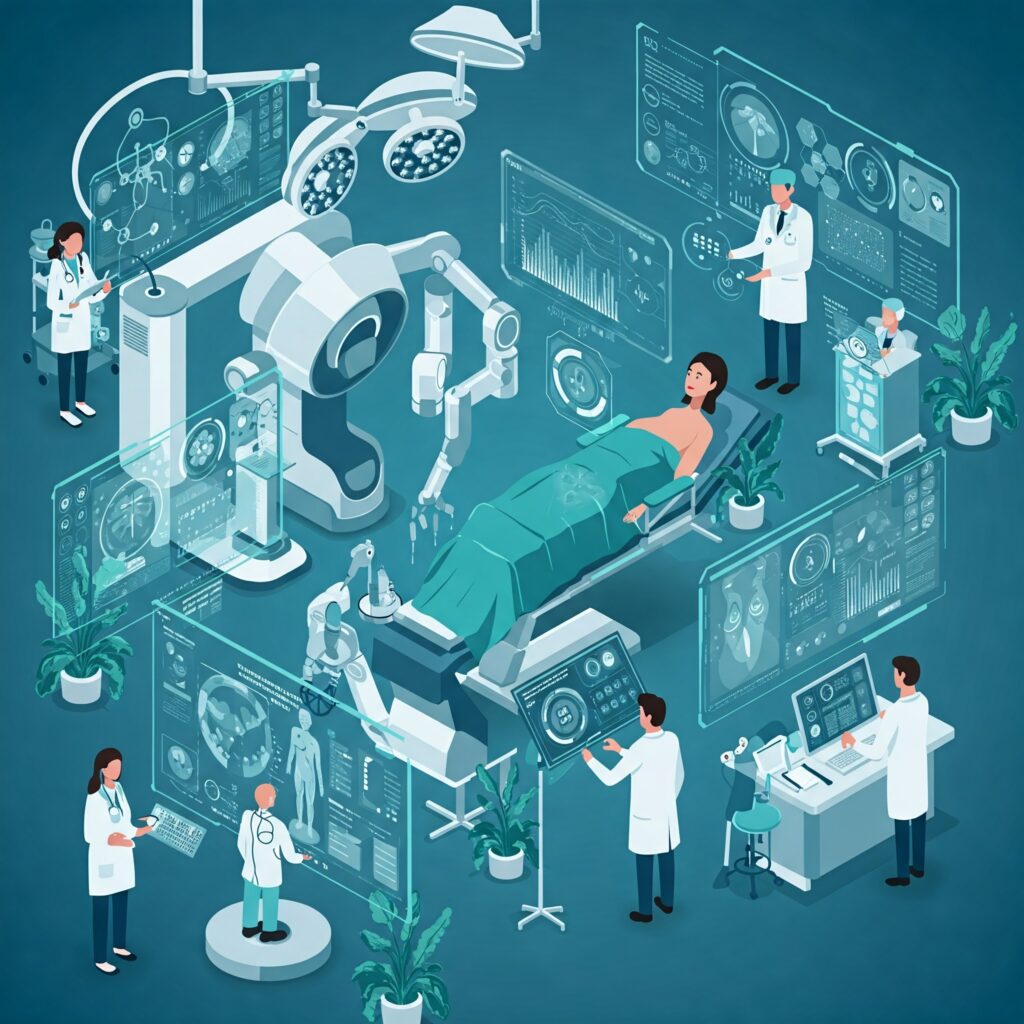
2. AI-Assisted Medical Imaging and Diagnostics
Medical imaging data, including X-rays, MRIs, and CT scans, is used to train deep learning models that can detect diseases faster and with greater accuracy than traditional methods. AI algorithms can analyze images to identify subtle abnormalities that might be missed by the human eye, leading to earlier and more accurate diagnoses.
Example: AI models developed by Tata Memorial Centre help identify early-stage lung and breast cancer in radiology scans, improving survival rates. By detecting cancer at an early stage, treatment options are more effective, and patient outcomes are significantly improved.
3. Personalized Medicine and Treatment Optimization
AI uses genomic data, EHRs, and patient histories to recommend personalized treatments based on an individual’s genetic profile and lifestyle factors. This approach ensures that each patient receives a treatment plan tailored to their unique biological makeup, increasing the likelihood of successful outcomes and reducing adverse effects.
Example: AI-driven precision medicine tools in hospitals like Medanta and AIIMS Delhi analyse patient data to suggest personalized cancer treatments based on genetic markers. This personalized approach to medicine is revolutionizing cancer care by making treatments more effective and less invasive.
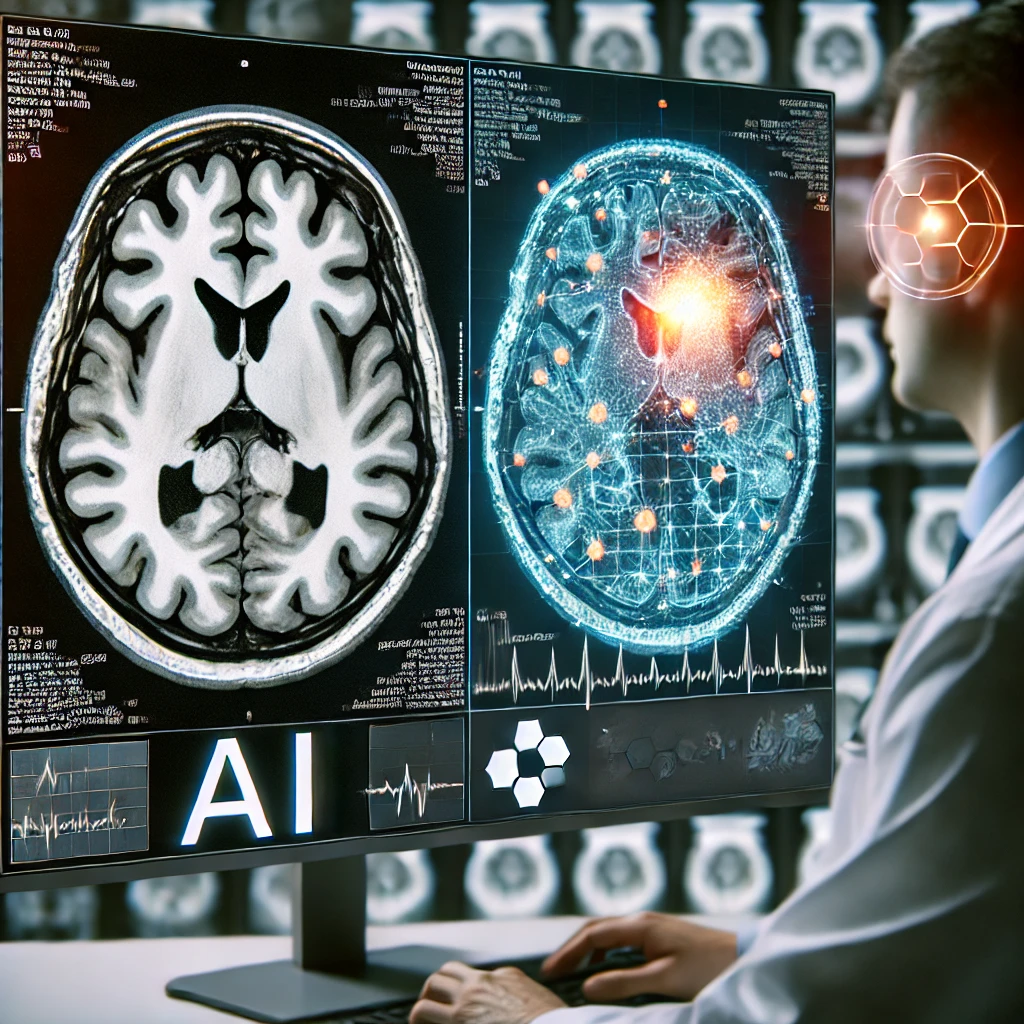
4. AI in Drug Discovery and Clinical Trials
Pharmaceutical companies use AI and Natural Language Processing (NLP) to analyse medical literature, clinical trial data, and patient histories to accelerate drug discovery and optimize trial designs. AI can identify potential drug candidates, predict their efficacy, and streamline the clinical trial process, making it faster and more cost-effective.
Example: Google’s DeepMind has used AI to predict the 3D structure of proteins, revolutionizing drug development for diseases like Alzheimer’s. By understanding the structure of proteins, researchers can design drugs that target specific molecular pathways, leading to more effective treatments.
5. AI-Powered Virtual Health Assistants and Chatbots
Conversational AI models trained on patient data, medical records, and symptom databases are now assisting telemedicine services and guiding patients with self-care and medication adherence. These AI-powered assistants can provide real-time medical advice, answer patient queries, and offer support between clinical visits, improving patient engagement and outcomes.
Example: AI chatbots like Practo and Mfine provide real-time medical advice by analysing symptom data and medical history. These chatbots can help patients manage their health more effectively by providing timely information and support.
6. Remote Patient Monitoring and Wearable Data Analysis
Smart wearables and remote health monitoring devices generate real-time health data that AI models analyse to detect anomalies and alert patients or doctors. This continuous monitoring allows for early detection of health issues, enabling proactive interventions and improving patient outcomes.
Example: Wearable devices like GOQii and BeatO monitor chronic diseases like diabetes, providing AI-powered alerts for abnormal blood sugar levels. By keeping track of health metrics in real-time, these devices help patients and healthcare providers manage chronic conditions more effectively.
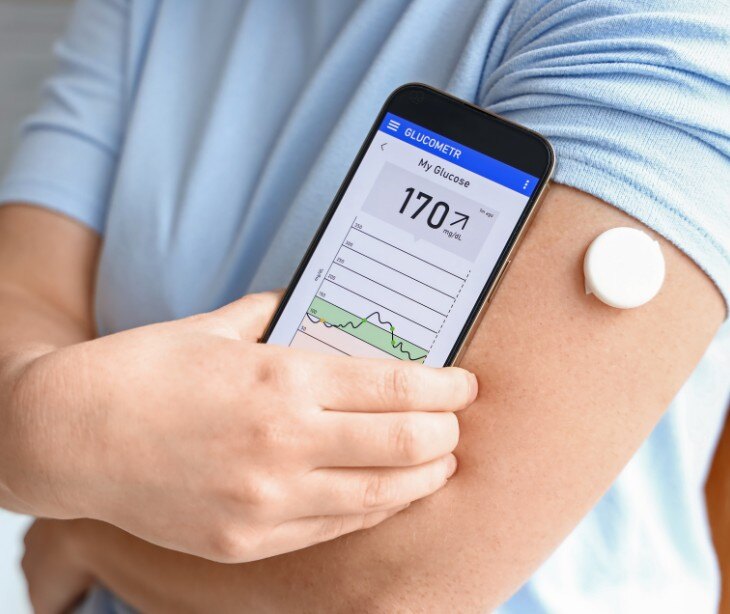
Standardizing Health Data for AI: Global and Indian Frameworks
For AI to deliver reliable and ethical healthcare solutions, structured and standardized medical data is essential. Key global and Indian health data standards include:
1. DICOM (Digital Imaging and Communications in Medicine)
· Standardizes storage, transmission, and display of medical images.
· Ensures interoperability across imaging systems like MRI, CT scan, and ultrasound machines.
Example: Teleradiology firms in India use DICOM standards to enable remote diagnosis through cloud-based imaging solutions. This allows healthcare providers to access and interpret medical images from anywhere, improving diagnostic accuracy and efficiency.
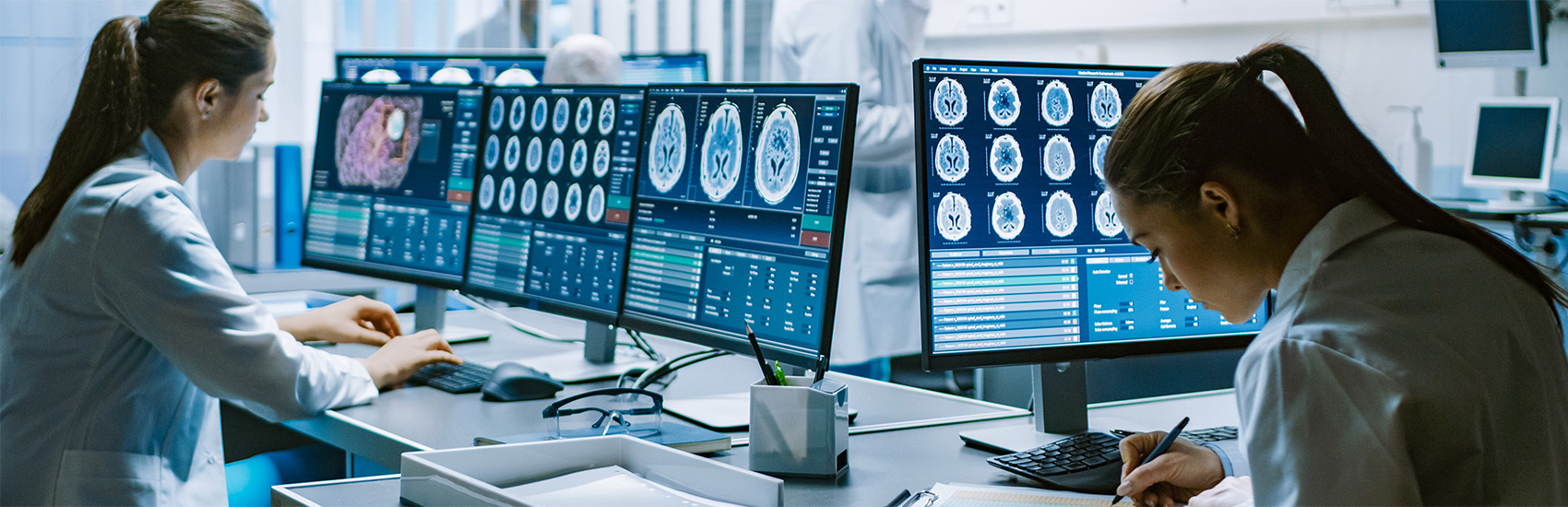
2. HL7 (Health Level Seven International)
· Facilitates seamless data exchange between healthcare providers, insurance firms, and pharmacies.
· Used in hospital management systems to integrate clinical applications.
Example: India’s Ayushman Bharat Digital Mission (ABDM) is adopting HL7 standards to enable a nationwide interoperable health system. This ensures that healthcare data can be easily shared and accessed across different healthcare providers, improving coordination and continuity of care.
3. SNOMED CT (Systematized Nomenclature of Medicine – Clinical Terms)
· Standardizes clinical terminology for accurate disease classification and AI training.
· Essential for NLP-based clinical decision support systems.
Example: AIIMS is integrating SNOMED CT to create an AI-powered clinical diagnosis assistant. This helps in standardizing medical terms used in patient records, making it easier for AI models to understand and analyse clinical data.
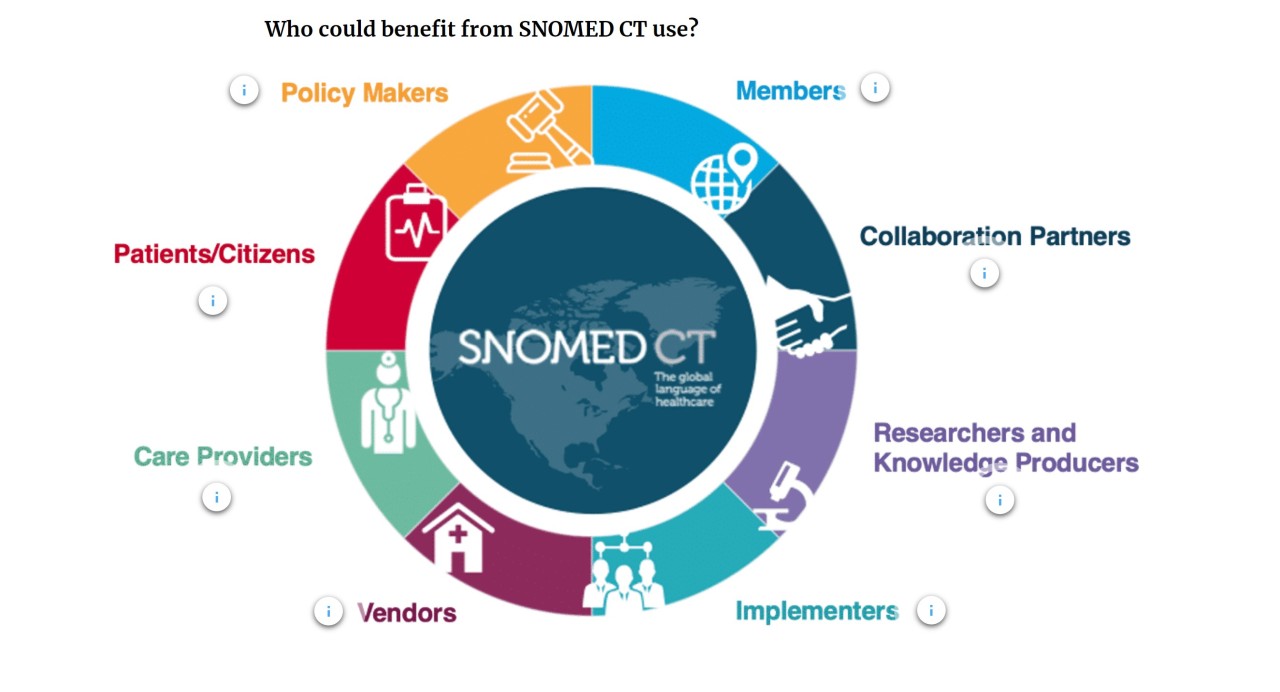
4. FHIR (Fast Healthcare Interoperability Resources)
· Enables web-based health data sharing using APIs.
· Powers telemedicine platforms and mobile health applications.
Example: Google Health and Apple Health leverage FHIR APIs to allow users to access their medical data securely. This enables patients to have control over their health data and share it with healthcare providers as needed, enhancing patient engagement and care coordination.
5. LOINC (Logical Observation Identifiers Names and Codes)
· Standardizes lab test results across hospitals and diagnostic centers.
· Ensures accurate and consistent reporting of medical data.
Example: Apollo Hospitals uses LOINC to integrate lab results into their AI-driven diagnostic systems. This standardization helps in maintaining consistency in lab test results, making it easier for AI models to analyse and interpret the data.

6. ICD (International Classification of Diseases)
· Standardizes disease classification for global epidemiology, health management, and clinical decision-making.
· Ensures consistent coding and reporting of medical conditions.
Example: ICD codes are used in Ayushman Bharat health claims processing and hospital billing systems. This ensures that diseases are classified consistently across different healthcare providers, facilitating better data analysis and reporting.
7. CPT (Current Procedural Terminology)
· Standardizes billing and documentation of medical procedures.
· Ensures accurate and consistent coding for medical services.
Example: Apollo Hospitals uses CPT for standardized billing and documentation of medical procedures. This helps in maintaining consistency in how medical procedures are documented and billed, making it easier to analyse and manage healthcare costs.
8. RxNorm
· Provides normalized names for clinical drugs and links its names to many of the drug vocabularies commonly used in pharmacy management and drug interaction software.
Example: RxNorm is used in electronic prescribing systems to ensure accurate and standardized drug information, reducing medication errors. This helps in maintaining consistency in how drugs are named and described, making it easier for AI models to analyse and interpret prescription data.
9. NCPDP (National Council for Prescription Drug Programs)
· Provides standards for pharmacy services, including electronic prescribing, billing, and medication history.
Example: NCPDP standards are used in pharmacy management systems to ensure accurate and efficient processing of prescriptions and billing information. This helps in maintaining consistency in how pharmacy services are managed, making it easier for AI models to analyse and interpret pharmacy data.
10. OMOP (Observational Medical Outcomes Partnership)
· A standard for representing healthcare data in a consistent format, facilitating the analysis of observational data from different sources.
Example: OMOP is used in research and analytics to integrate and analyse healthcare data from various sources, enabling large-scale observational studies. This helps in maintaining consistency in how healthcare data is represented, making it easier for AI models to analyse and interpret the data.
Ensuring Data Security in AI-Driven Healthcare
AI-powered healthcare applications require strong security frameworks to protect patient data from breaches, unauthorized access, and cyber threats. Key security frameworks include:
1. HIPAA (USA – Health Insurance Portability and Accountability Act)
· Ensures confidentiality, encryption, and access control for patient data.
· AI models handling EHRs or telemedicine services must comply with HIPAA to prevent data misuse.
2. GDPR (EU – General Data Protection Regulation)
· Protects personal health data and gives patients control over their records.
· AI-driven medical services must ensure explicit patient consent before processing health data.
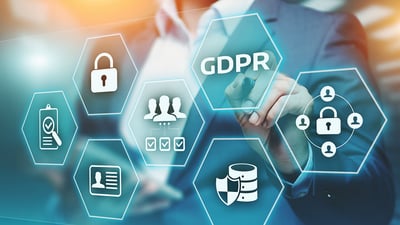
3. India’s Digital Personal Data Protection (DPDP) Act, 2023
· Governs the collection, processing, and sharing of personal health data.
· Mandates data encryption, secure storage, and patient consent management.
Example: Under DPDP, hospitals using AI-driven patient analytics must obtain explicit consent before processing medical records. This ensures that patient data is handled ethically and securely, protecting patient privacy and building trust in AI-driven healthcare solutions.
4. Ayushman Bharat Digital Mission (ABDM) Data Privacy Framework
· Establishes secure health record storage and patient-controlled data access.
· Uses blockchain and decentralized storage to minimize data breaches.
Example: ABDM’s Ayushman Bharat Health Account (ABHA) ID allows patients to securely access their health records across different hospitals. This ensures that patient data is stored securely and can be accessed only with patient consent, enhancing data privacy and security.

Conclusion: The Road Ahead
AI-driven healthcare solutions rely on high-quality, standardized health data to improve patient outcomes, streamline medical processes, and enhance research. By adopting global and Indian health data standards, ensuring robust security measures, and prioritizing ethical AI practices, we can unlock the full potential of AI in revolutionizing healthcare.
With India’s push toward digitized and AI-powered healthcare under ABDM, the future holds immense potential for personalized medicine, predictive analytics, and improved patient care.
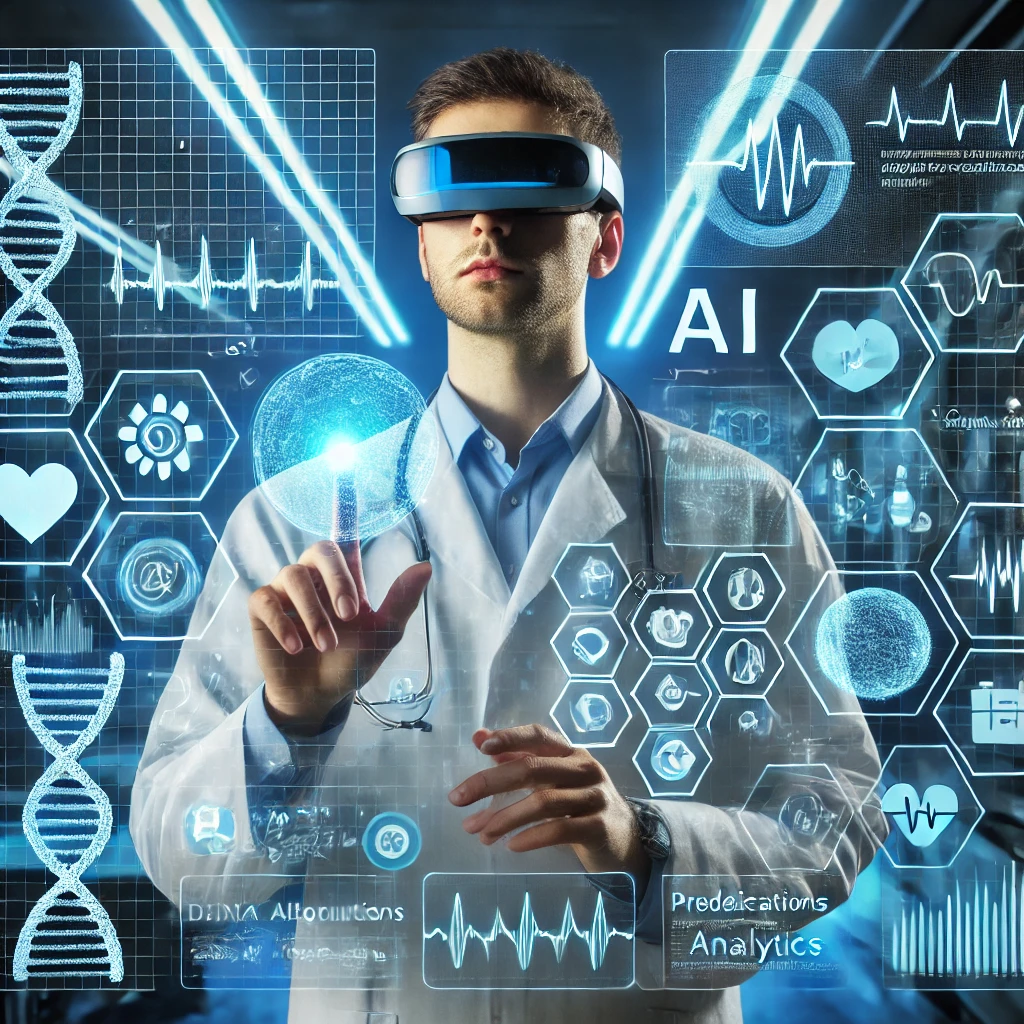